Predictive HR Analytics: Transforming Workforce Management
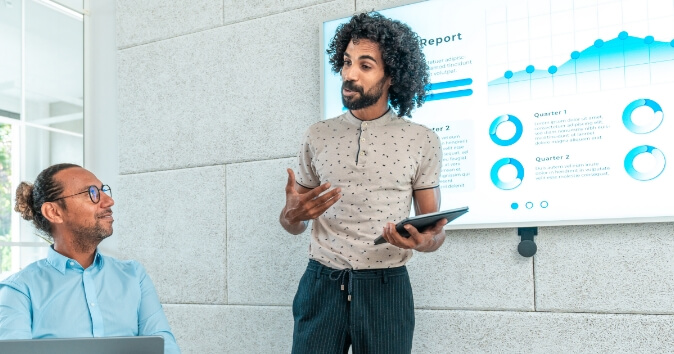
Wouldn't it be fun if we could peer into the future? Enter predictive HR analytics, an AI capability making waves across the HR industry by allowing you to get ahead of future issues and tackle them before they even materialize.
A report by PR newswire shows that Deloitte principal Josh Bersin says that "only 17% of organizations worldwide have accessible and beneficial HR dataOpens in a new tab” That leaves a whole lot of companies guessing at where to put time, resources, and money. Read on to learn about predictive HR analytics, its benefits, how to implement it successfully, and more.
What is predictive HR analytics?
Predictive HR analytics takes historical data and analyzes it using machine learning techniques and statistical algorithms to make predictions on workforce future trends and behaviors.
Its purpose is to help HR professionals take a proactive stance. You’re using data to anticipate potential challenges and opportunities within the organization that may arise before they happen. This affords you the ability to correct before issues arise.
Predictive analytics provide valuable insights that:
- Help optimize recruitment by improving recruitment practices
- Lead to improvements in performance management
- Remove the guesswork by providing you with data-driven decisions
- Make it easy to create employee profiles that distinguish who will thrive and who will not in your organization
This means you can better allocate resources and anticipate important metrics like turnover rates. Predictive HR analytics also helps in identifying emerging trends before they become obvious, providing a competitive advantage for companies.
When it comes to analytics, there are several types that can support HR functions. Let's explore what they are together.
How does predictive analytics differ from descriptive analytics?
The primary difference between predictive and descriptive analytics lies in their focus and purpose.
Descriptive analytics is about understanding past performance by analyzing historical data. It answers the question, "What happened?" Diagnostic analytics takes this further by exploring why something happened, providing insights to help avoid similar issues in the future.
HR predictive analytics, on the other hand, shifts the focus to future outcomes. It uses historical HR data and advanced statistical models powered by machine learning to anticipate what is likely to happen.
This type of analysis enables organizations to forecast future workforce trends, allowing them to plan proactively and mitigate potential risks. Additionally, predictive analytics empowers businesses to seize opportunities in a forward-thinking, strategic manner.
Prescriptive analytics goes one step beyond prediction, recommending specific actions to achieve desired outcomes based on the forecasts.
Benefits of HR predictive analytics
The strategic advantage lies in actionable insights that can have a big impact on the HR department. Let’s take a look at some of the top areas predictive HR analytics can improve:
Improved hiring decisions
Analyzing data in advance with accurate predictive modeling can optimize your hiring process by identifying patterns in important factors like job performance or what channels produce the best candidates. This can answer questions like:
- What candidates have the capacity for long-term success?
- Who will be more likely to become top performers?
- How can X process be streamlined without compromising quality?
Enhanced employee retention strategies
A predictive analytics model can forecast when employees are most likely to check out and what factors play a role in it, including:
- Demographics
- Types of roles
- Departments
- Job satisfaction levels
With this information in the HR toolkit, you can implement targeted retention strategies that put an end to high turnover, such as:
- Personalized development programs
- Work-life balance initiatives
- Recognition and reward systems like Social Recognition® from Workhuman®
Workhuman is the #1 provider of employee recognition software. When you opt for recognition done right from Workhuman, you don't have to settle for second-rate recognition.
Increased workforce productivity by optimizing performance
Performance optimization is a cornerstone of workforce productivity. You want the best from your employees, which requires understanding what motivates them and where skill gaps hinder their success.
Modeling team and individual performance metrics can tell you:
- What areas of performance need improvement
- Which employees would benefit most from targeted training
- How roles can be tailored to maximize individual contributions
Predicting what will be needed at what point in the employee lifecycle will help you intervene with strategies that align tasks with an employee's capabilities. You can provide personalized development plans that promote growth and engagement.
You will also be able to identify what specific tools may be useful to help drive better results by enabling employees to work more efficiently and effectively.
Effective engagement strategies for better employee satisfaction
An engaged workforce is a happier workforce. This can show up in metrics like a drop in absenteeism and turnover rates.
Predictive modeling can show you:
- What areas of engagement need improvement
- Which strategies resonate most with employee needs
- How you can best address disengagement
It is also a great way to forecast how employee engagement will impact your sales.
Armed with these kinds of insights, you can design tailored strategies that empower employees to do their best, improving morale and satisfaction in the process.
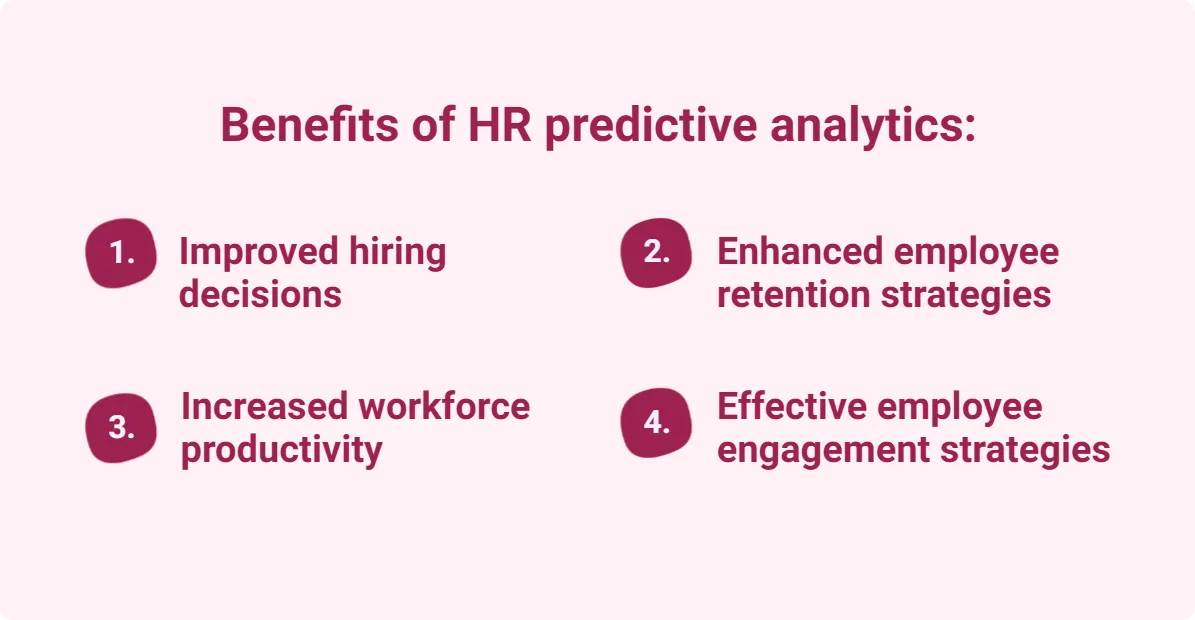
Steps to successfully implement predictive analytics in HR
Research by the Rand Corporation states that over 80% of AI projects fail. How do you be part of the 20%? Here are some useful steps to set you up for success:
Define clear objectives
Creating awareness of the challenges your HR team is facing will guide you on what goals to set. You can look at this as the first step and combine it with longer-term objectives that define what success looks like.
Typical challenges in HR include:
- Adapting to remote and hybrid work
- Managing diversity and inclusion
- Integrating technology
Remember to create goals with precise metrics you can use to measure your performance. This will make it easier to keep projects in line with your organization's broader objectives.
Clear objectives look like:
- Reduce time to hire by 20%
- Achieve a 15% improvement in Net Promoter Score (NPS)
- Improve leadership pipeline readiness to 60%
Assemble a competent team
It will be smoother to integrate AI by establishing structures that promote collaboration. A cross-functional team of HR professionals and data analysts or scientists is essential for aligning AI projects with business goals.
You could also get your HR personnel up to speed on how to use and interpret predictive insights effectively by providing data literacy training.
Gather and prepare historical data
AI’s performance relies heavily on the quality of the data it processes. So, when you gather relevant data from systems like HRIS and ATS, it’s important to clean it. This will provide consistent data that can generate accurate results and reliable insights.
Handling data properly will also help you stay compliant with data privacy regulations, such as GDPR or CCPA.
Choose the right tools and technology
When you know your main objectives and what will be required for success, you will have a clearer idea of what tools you need.
It's important to factor in your legacy systems when you choose what predictive HR analytics tech to implement. SAP SuccessFactors and Oracle HCM Cloud are widely used in HR. Platforms like this commonly deploy tools like:
- Data visualization that breaks down complex data for easy interpretation
- Sentiment analysis that gauges employee mood and satisfaction
- Workforce planning and scenario modeling that can simulate various workforce scenarios
Machine learning in HR analytics includes plenty of invaluable techniques, such as regression analysis and decision trees:
- Regression analysis predicts quantitative outcomes and quantifies relationships, which is great for numeric results like compensation modeling.
- Decision trees segment data into subsets based on decision rules, making them easier to interpret for HR analytics. They simplify complex decisions into tree-like structures, showing paths to various outcomes. This is valuable for turnover risk classification or succession planning.
Depending on how mature your business is, there will be different considerations when you choose a solution, such as scale, budget, and technical capabilities. It’s important to choose a scalable solution that can grow with your business.
Here are some general guidelines:
- Start small with a pilot initiative, focusing on a specific HR function like retention.
- Gather feedback and refine models before expanding.
- Test, proof, and integrate them into HR workflows and monitor their performance to ensure continuous improvement.
- Measure ROI by quantifying the impact on KPIs. Share results with stakeholders through clear reports and dashboards.
- Continuously update models with new data to keep them relevant and effective.
Develop predictive models
Start by using historical data to train algorithms and identify patterns like turnover or engagement. Focus on relevant metrics to align models with organizational priorities. Validate the models to ensure predictions are accurate and actionable.
Involve stakeholders
Engage stakeholders early on, which will be vital for you to gain support and align predictions with organizational goals. This will help you secure buy-in from HR leaders, managers, and employees, who will, in turn, help you create relevant models.
Pilot the initiative
Test the models in a small, specific HR function before expanding. Take feedback from the pilot phase and iterate to prove their effectiveness for broader implementation.
Deploy and monitor
Once the models are tested, integrate them into HR workflows and monitor their performance so they remain effective. Allowing for adjustments will generate continuous improvement.
Measure and communicate ROI
Quantify the impact of predictive analysis through KPIs such as turnover reduction and improved hiring outcomes. Use dashboards and reports to communicate these results to stakeholders and demonstrate the value of the initiative.
Continuously improve
Regularly update models with new data to maintain their accuracy and relevance. Stay informed about advancements in HR predictive analytics to improve models and keep them aligned with best practices.
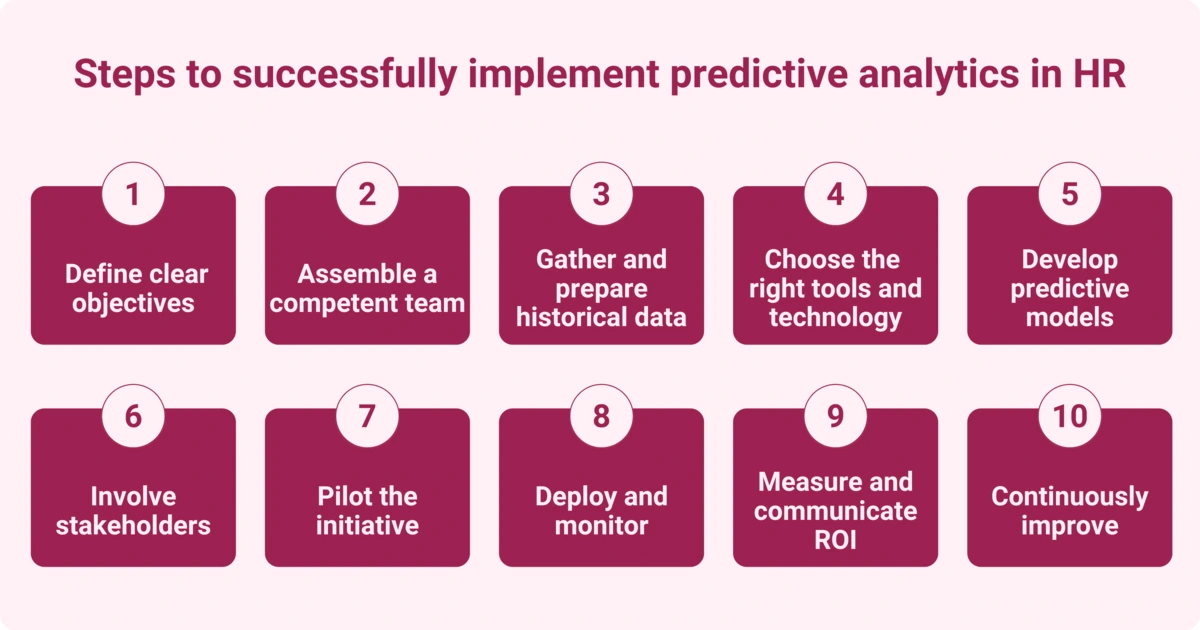
Challenges in implementing predictive HR analytics
While your HR teams will advance by implementing predictive analytics, creating a winning solution comes with obstacles you need to overcome.
1. Addressing data privacy concerns in HR analytics systems
AI relies on massive amounts of data to function effectively, which invites major privacy and security concerns. To protect data, organizations must establish strong privacy policies and comply with regulations like GDPR.
Companies must implement encryption, access controls, and monitoring systems to protect sensitive data from unauthorized access or malicious activity. A "data minimization" approach ensures that only necessary data is collected, maintaining privacy while enabling valuable insights.
2. Tackling biases in predictive algorithms to ensure fairness
AI model training data can inherit prejudices that reflect the unconscious biases of developers, and so can the usage data collected from users. This can sustain or intensify discrimination, leading to unfair or harmful outcomes, especially in areas like hiring or lending.
A strong ethical framework, along with regular audits, is necessary to identify biases in models and promote diverse, equitable outcomes. HR teams can mitigate this by:
- Rebalancing datasets
- Using fairness-aware machine learning techniques and diversity and inclusion analytics tools
3. Overcoming integration challenges of disparate data sources
Integrating data from multiple systems (HRIS, ATS, payroll, etc.) can be complex due to inconsistencies in formats and incomplete records.
Using specialized tools and implementing data governance practices helps streamline integration, making data compatible and consistent.
4. Ensuring transparency in data usage and employee trust
With many AI systems failing to provide explanations of how they reach certain conclusions, you can run the risk of operating systems in ways that act as "black boxes." This creates significant transparency issues. Complex models, like neural networks, often make decisions in ways not easily understood even by their creators.
Employing explainable AI tools creates transparency, which is critical for building trust and maintaining accountability.
It's good to have:
- Clear communication about how data is collected and used
- Explainable AI models that show exactly how decisions were made
- Feedback channels that address concerns
- Training to upskill employees
- AI champions who can promote how AI augments human work
Case studies and real-world applications
Some companies have reported strong results in their use of predictive HR analytics:
Successful implementation in recruitment processes
In Laszlo Bock’s book Work Rules, the former senior VP of people operations at Google reveals that statistics are at the top of how Google attracts and retains top employeesOpens in a new tab.
Google integrated a fully automated, computer-generated system into its hiring process that is fine-tuned by data analytics so it can identify the best candidates at speed.
Enhancing employee development programs with predictive insights
A Financial Times article reports that Johnson & Johnson uses an AI-driven process called skills interference that allows it to assess and plan in ways that would be impossible otherwise.
Trained to identify where skills already exist in the business, AI can tailor recommendations for learning and development that can complement and upskill employees.
Predicting employee flight risks to reduce turnover
In an interview on CNBC, IBM’s Gini Rometty said the company's predictive AI is now 95% accurate when forecasting an employee's plan to leave. This has saved IBM a whopping $300 million in retention costs.
Understanding data patterns and adjacent skills, IBM AI can zero in on an individual’s strengths, enabling managers to direct employees to opportunities that may not have been seen using traditional methods.
Future trends in predictive HR analytics
With artificial intelligence showing no signs of slowing down in the HR sphere, you can anticipate there will be continuous changes to come.
AI, machine learning, and improved data integration will shape the future of predictive people analytics. These technologies allow predictive models to evolve and refine themselves over time.
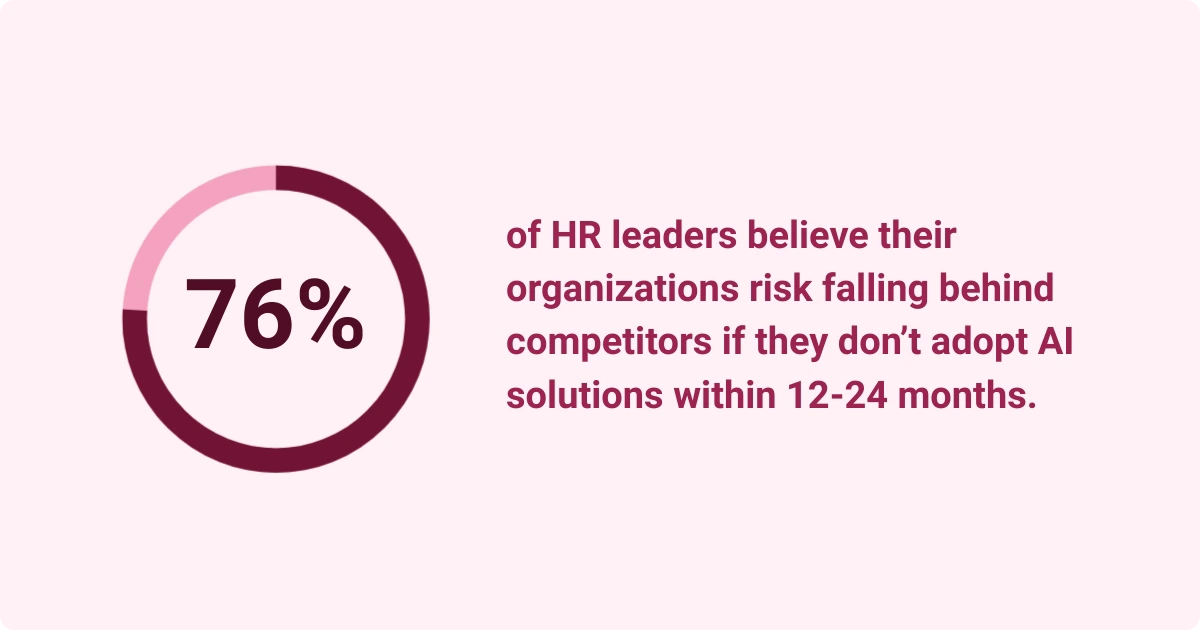
Some emerging human resource technology trends to watch out for in 2025:
- Use of Natural Learning Processing (NLP) to analyze employee feedback
- Increased focus on employee experience
- The prioritization of security alongside AI innovation
Conclusion
You can become a much more competitive business when you take advantage of technology like Workhuman IQ to get data-rich answers to HR questions. Sizable opportunities and savings can be found by taking proactive approaches to your HR activities.
Workhuman is a leading talent intelligence platform that takes skills management to the next level. With AI-powered Human Intelligence™ and skills mapping, it enables you to monitor workforce capabilities over time and measure the impact of upskilling and reskilling efforts.
Workhuman iQ goes even further, using AI-driven social analytics to turn raw data into actionable insights. It provides a deeper understanding of the employee experience, helping organizations make data-driven decisions with ease. It’s the kind of intelligence you’ve always wanted—delivered in a way anyone can use.
Predictive HR analytics are not only important – they are becoming a must to avoid falling behind.