5 Major Challenges of AI in 2025 and Practical Solutions to Overcome Them
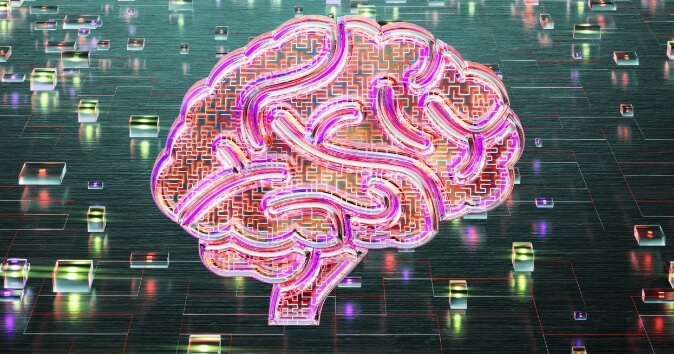
Artificial intelligence is on the rise, with no signs of slowing. According to an IBM report on AI spendingOpens in a new tab business executives plan to increase the use of artificial intelligence by 82% in 2025.
While this AI explosion comes with enormous possibilities, it also brings growing ethical concerns, from transparency issues to algorithm biases.
In this article, we’ll explore major challenges of AI in 2025, how you can implement measures to use AI responsibly, and how generative AI is shaping the future of the human workplace.
Major challenges of AI on the horizon
The rapid adoption of current AI technologies across various business sectors, from accounting to analytics, raises significant concerns around ethical AI practices, particularly regarding the handling of personal and sensitive data. Data management remains a critical area of focus, as companies must balance leveraging vast amounts of data while ensuring regulatory compliance.
Protecting the privacy and security of employees and customers alike is crucial to maintaining trust. As AI systems increasingly make decisions that impact individuals' lives, there is more potential for:
- Misuse
- Bias
- Data breaches
Ensuring security and protecting your people remains crucial in the ethical use of AI. Policies that foster transparency and enforce robust data protections help preserve individuals’ rights and wellbeing while confronting both immediate and long-term risks associated with AI.
1. Ethical concerns in AI
As AI becomes more integrated into daily life, it raises ethical dilemmas from fairness in decision-making to privacy. Let’s discuss the major ethical challenges you might face when working with AI and practical steps to overcome them.
Accountability issues
Assigning responsibility for AI-driven decisions is a complex challenge. When AI systems make mistakes or cause harm, it is difficult to determine whether developers, operators, or the AI itself is at fault. This lack of clarity complicates legal and ethical frameworks, making it hard to enforce accountability.
Lack of transparency
With many AI systems failing to provide explanations of how they reach certain conclusions, you can run the risk of operating systems in ways that act as "black boxes." This creates significant transparency issues. Complex models, like neural networks, often make decisions in ways not easily understood even by their creators.
Transparency is critical for building trust and maintaining accountability. This is especially important across high-stakes domains such as healthcare, law enforcement, and finance, where the consequences of AI decisions can profoundly impact lives.
Ethical AI frameworks
Creating ethical AI frameworks is essential to the responsible development and deployment of AI technologies. These frameworks should address key ethical considerations:
- Diversity: Training AI models on datasets that reflect a wide range of demographic, social, economic, and cultural contexts is crucial for minimizing bias and having fair outcomes. Regular audits, tests, and tools like fairness-aware machine learning can help identify and correct biased outcomes so AI systems operate equitably.
- Inclusivity: Incorporating inclusive design practices and involving diverse teams of developers and stakeholders throughout the development process is another key aspect of ethical AI. This helps reduce bias at every stage.
- Transparency: Employing explainable AI tools allows for greater transparency in decision-making processes, making it easier to detect and rectify any unintended biases. Ethical AI frameworks provide the necessary structure to guide these efforts and build trust in AI systems so they are used responsibly.
AI bias
AI model training data can inherit prejudices that reflect the unconscious biases of developers. So can the usage data collected from users. This can sustain or intensify discrimination, leading to harmful or unfair outcomes, especially in areas like hiring or lending.
There are two primary types of bias in AI:
- Unconscious (implicit) bias: A hidden form of bias that operates subconsciously, often disguised as intuition, influencing decisions without our awareness
- Conscious (explicit) bias: A visible and deliberate form of bias that we recognize, justify, and sometimes embed into systems and practices
Unconscious bias can be prevalent in the workplace, where a lack of diversity in AI can turn into learned biases and reinforce existing inequalities. For example, an AI recruiting tool could exhibit unconscious bias by favoring male candidates if its training data reflects historical gender discrimination.
In MIT Media Lab's "Gender Shades: Intersectional Accuracy Disparities in Commercial Gender Classification" report, Joy Buolamwini and Timnit Gebru found that facial recognition software often performs poorly on darker skin tones due to underrepresentation in training datasets. These biases erode trust in AI and can cause real-world harm.
In content, conscious bias can create feedback loops, targeting or isolating specific groups, feeding the same content repeatedly. It can blind users from new or diverse information and reinforce political stances that polarize society.
By actively identifying and eliminating bias, AI can become a tool for equity rather than inequality. This approach to AI is another way to build trust and make sure AI systems serve everyone fairly.
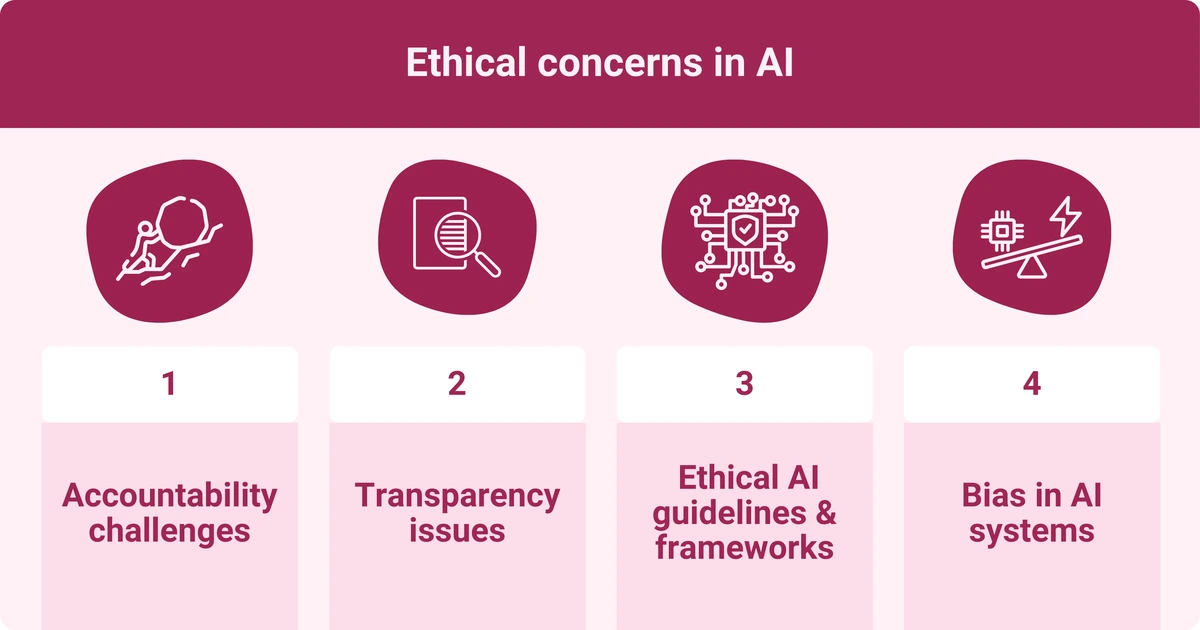
2. Data privacy and security concerns in AI applications
AI relies on massive amounts of data to function effectively, which invites major privacy and security concerns.
Data security challenges
AI systems often process vast quantities of sensitive data, making them attractive targets for cyberattacks. Hackers may exploit vulnerabilities in AI algorithms or storage systems to steal confidential information, such as financial details or healthcare records.
For instance, a data breach in an AI-powered healthcare system could expose patient histories, leading to identity theft or other harm. Companies must implement robust encryption methods, access controls, and monitoring systems to protect sensitive data from unauthorized access or malicious activity.
Safety and security risks in AI applications
Many artificial intelligence applications collect and track personal data, often without users fully understanding the privacy risks associated with how their information is being used. For example, location-based AI services or social media recommendation systems may inadvertently expose users to profiling or targeted surveillance.
Without transparent policies and safeguards for AI, individuals risk losing control over their personal information. This leaves companies vulnerable to legal penalties and reputational harm.
Informed consent issues
Artificial intelligence systems frequently process user data without obtaining clear, informed consent. Users may not be aware of the extent of data collection or how their information will be analyzed and shared.
For example, smart home devices powered by AI might gather audio or video data without providing adequate notice. This lack of AI transparency erodes trust and raises ethical questions about user autonomy. Clear communication and consent protocols are essential to address these issues.
Surveillance and monitoring
AI’s ability to analyze data in real time makes it a powerful tool for surveillance and monitoring. While this has legitimate uses, such as protecting public safety, it also raises concerns about misuse.
For instance, AI-powered facial recognition technology has been deployed in ways that enable mass surveillance, disproportionately affecting marginalized groups. Balancing the benefits of AI in security with individuals’ rights to privacy is a critical challenge for regulators and developers.
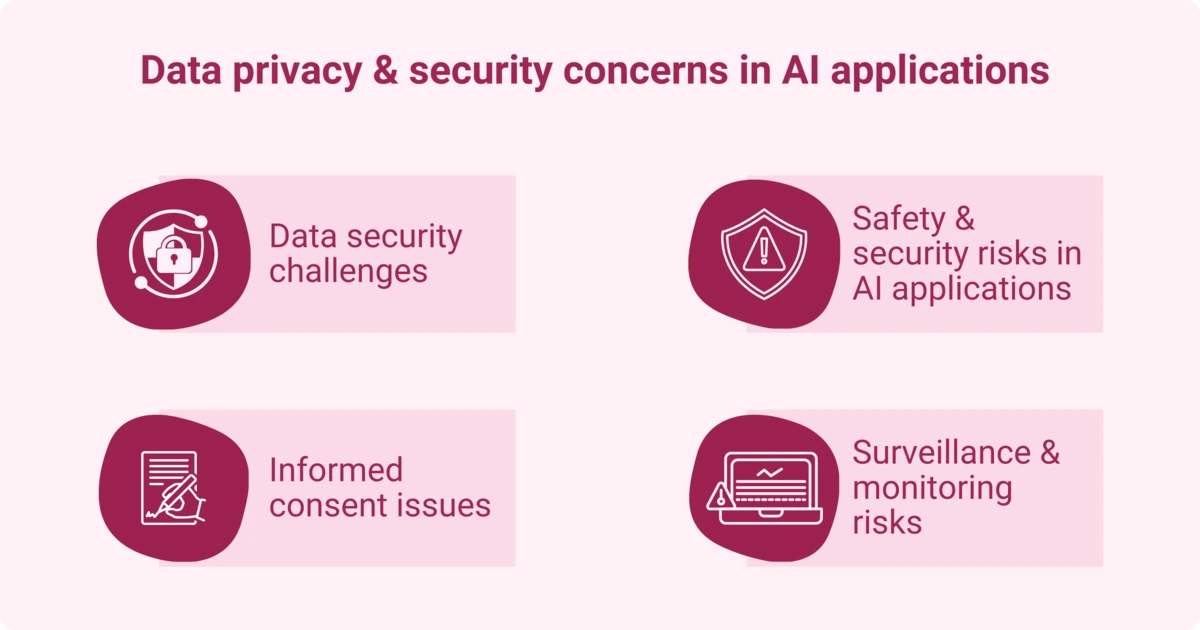
3. Technical barriers to AI integration and scaling
The widespread adoption of AI is often hindered by technical challenges that complicate its integration and scaling. For example, AI systems must often interact with legacy infrastructure, which may lack the compatibility to support advanced AI capabilities.
Furthermore, scaling AI for tasks like real-time data analysis requires significant computational resources and careful optimization of algorithms to avoid inefficiency. Without addressing these barriers, many organizations struggle to achieve the full potential of AI in their operations.
AI model training challenges
Training AI models effectively is a complex process that depends heavily on the quality and quantity of data. Bad data quality, such as missing values or unbalanced datasets, can lead to models that make inaccurate predictions or reinforce artificial intelligence biases.
For instance, facial recognition systems trained on datasets lacking diversity have produced discriminatory results. Overfitting, where models perform well on training data but poorly on new data, is another significant issue. Insufficient training data or errors in preprocessing can further compound these data related challenges, creating unreliable AI solutions.
Scalability and efficiency of AI systems
Scaling AI systems for large-scale applications often exposes limitations in computational power and algorithm efficiency. Real-time applications, such as traffic monitoring and fraud detection, require immense processing capabilities and fast response times.
AI systems deployed in cloud environments may face high memory usage or bottlenecks in data transfer speeds. For example, deep learning models used in image recognition can be computationally intensive, necessitating hardware upgrades and optimization techniques to handle increasing workloads efficiently.
Integration with existing systems
Incorporating AI into legacy systems presents significant obstacles. Many infrastructures lack the compatibility or processing capacity needed for AI solutions.
For instance, manufacturers seeking to use predictive maintenance powered by AI often need to retrofit older machines with sensors and networking capabilities. This process requires not only financial investment but also time and technical expertise, making integration a challenging endeavor for many organizations.
Lack of skilled professionals
The demand for skilled AI professionals far exceeds the available talent pool, creating bottlenecks for organizations aiming to develop and deploy AI solutions.
There are significant shortages of professionals to fill roles in:
- Data science
- Engineering
- AI implementation and prompting
This means companies often face delays in implementing AI projects or compromise on the quality of their solutions.
For instance, a lack of expertise in natural language processing can result in underperforming chatbots or voice assistants, limiting their usability. This talent gap is a major impediment to AI’s widespread adoption.
Infrastructure limitations
AI development and deployment are often constrained by inadequate computing infrastructure. Resource-intensive tasks, such as training large language models, require specialized hardware like GPUs or TPUs, which may not be available in many organizations.
In resource-constrained environments, such as rural areas or developing countries, limited storage capacity and unreliable network connections further restrict AI applications. These infrastructure gaps can delay the development of critical AI solutions, such as medical diagnostic tools or agricultural AI systems.
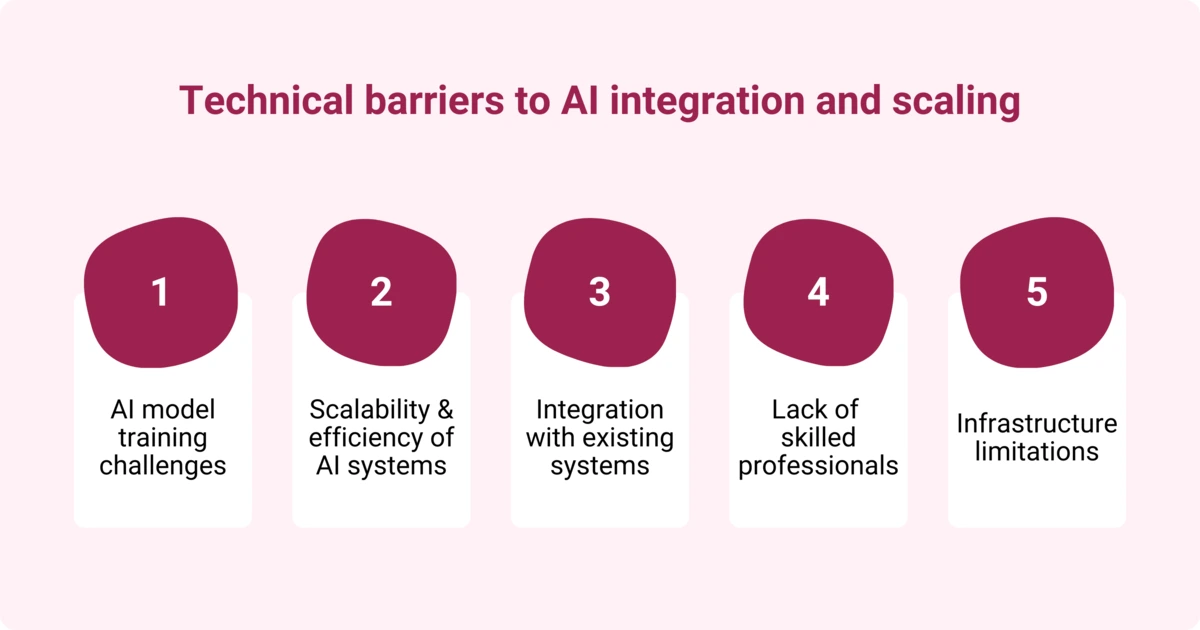
4. Legal challenges in AI
As AI systems take on more autonomous roles in decision-making, determining legal accountability and ownership rights becomes increasingly difficult. Governments and organizations are now grappling with creating legal structures surrounding AI regulation that keep pace with the rapid evolution of AI technologies.
Legal liability in AI deployment
Determining legal liability in AI-related incidents is a significant challenge. When AI systems malfunction or make harmful decisions, it is often unclear who is responsible:
- The developer
- The operator
- The end-user
For example, if an autonomous vehicle causes an accident, assigning liability could involve multiple parties, including the AI software developer, the car manufacturer, and the person overseeing the system. This ambiguity complicates legal proceedings and raises questions about the ethical implications and accountability in AI deployment.
Intellectual property rights
AI introduces complex questions about intellectual property ownership. For instance, when AI generates original content – such as artwork, music, or inventions – it remains unclear who holds the rights to these creations:
- The developers of the AI
- Its operators
- No one at all
Additionally, patenting AI-driven innovations faces challenges, as current laws are often ill-suited to handle technologies that evolve autonomously. Legal disputes over AI-generated content are becoming more frequent, highlighting the urgent need for updated IP frameworks.
Emerging regulatory frameworks
As AI advancements proliferate, governments are beginning to implement laws and regulations to govern their development and use.
For example, the European Union's AI ActOpens in a new tab addresses issues ranging from transparency to safety in high-risk AI applications. However, creating adaptable regulatory frameworks that can keep pace with rapid technological advancements is challenging. They must strike a balance between encouraging innovation and mitigating risks.
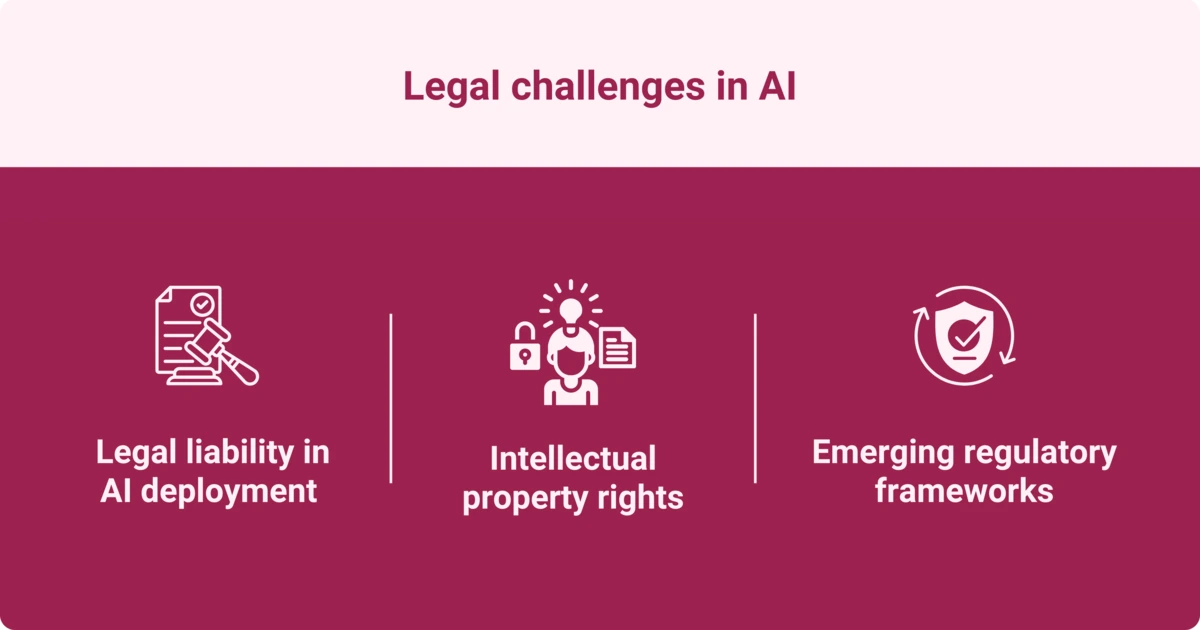
5. Economic and social consequences of AI adoption
AI has the potential to disrupt labor markets and exacerbate inequalities in societies. It can even manipulate equitable access to technology's advantages.
Job displacement due to AI automation
AI-driven automation is poised to displace jobs across many industries, such as:
- Manufacturing
- Customer service
- Transportation
- Retail
- Financial services
- Creative industries
- And more
Autonomous vehicles threaten to replace truck drivers, while AI-powered chatbots reduce the demand for customer support representatives. This shift could result in significant unemployment, especially for workers in repetitive or manual roles.
To combat this, you might consider reskilling programs to prepare workers for jobs in emerging fields, such as AI development and data analysis. This can help them adapt to a rapidly evolving job market.
Economic inequalities exacerbated by AI
AI adoption risks deepening economic inequalities by disproportionately benefiting large corporations with the resources to invest in advanced technologies. Smaller businesses, which often lack access to similar AI capabilities, may struggle to compete. This may widen the gap between large enterprises and smaller players.
Additionally, AI systems trained on biased data can perpetuate disparities in wealth and opportunity, such as denying loans to historically disadvantaged communities. Policymakers and organizations must address these issues by creating systems that promote the equitable distribution of AI’s benefits.
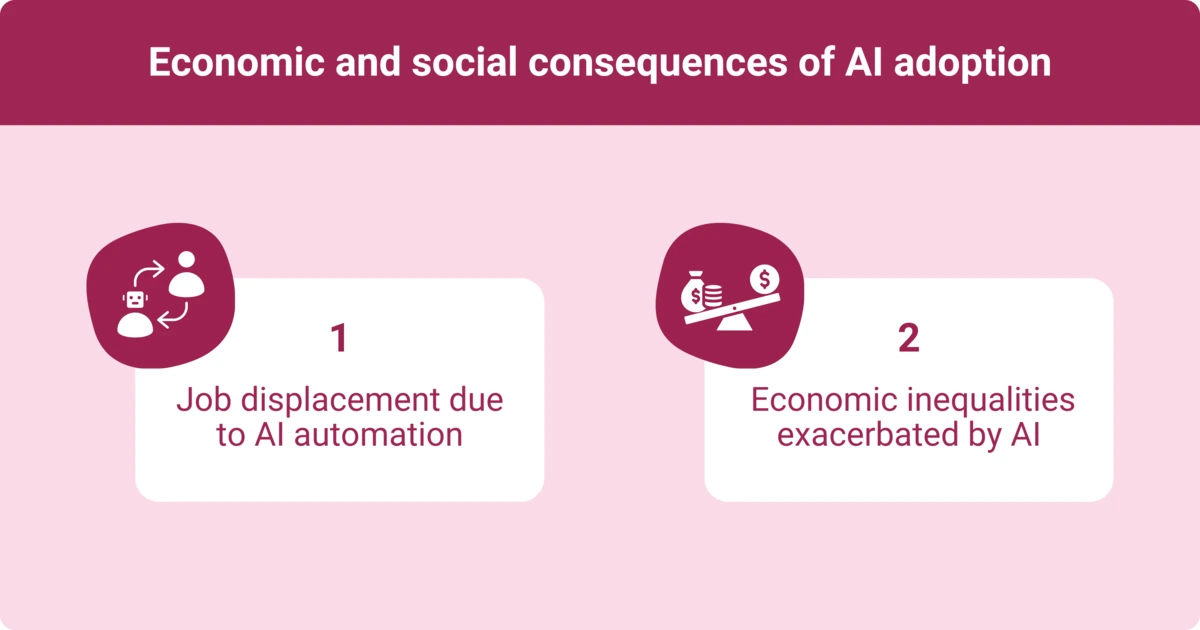
Building a sustainable framework for AI technologies
It’s important to create frameworks that make sure growth is both sustainable and responsible as well as aligned with societal needs.
Advancing data governance and quality control
Data governance plays a pivotal role in ensuring AI systems are built on accurate and ethically sourced data. Without strong data governance, AI models risk being trained on biased information that is outdated or incomplete.
Biased data could lead to flawed outcomes, such as a credit scoring algorithm unfairly denying loans to certain demographics.
Quality control processes, such as regular audits and the use of fairness-aware machine learning tools, help identify and mitigate these issues. Establishing clear data sourcing guidelines and implementing strict validation protocols are essential steps toward responsible AI development.
Broadening AI skillsets across industries
Skill mapping of employees and upskilling workers in AI is vital for addressing talent shortages and encouraging successful AI adoption across industries. Building AI competence might involve:
- Training employees in technical and non-technical roles that increase skill diversity in the workplace
- Enabling them to understand and apply AI tools effectively
- Promoting upskilling programs to empower workers to thrive in AI-enhanced environments
Harnessing tools to address your skills shortage head-on can unlock the full potential of AI while creating more opportunities for workers to thrive, increasing their job security and filling skill gaps in your organization. Workhuman® iQ is the perfect AI tool for gaining unparalleled views on things like people dynamics and skills that can lead to better employee retention and elevated ROI.
Workhuman is a leading talent intelligence platform that takes skills management to the next level. With AI-powered Human Intelligence™ and skills mapping, it enables you to monitor workforce capabilities over time and measure the impact of upskilling and reskilling efforts.
Workhuman iQ goes even further, using AI-driven social analytics to turn raw data into actionable insights. It provides a deeper understanding of the employee experience, helping organizations make data-driven decisions with ease. It’s the kind of intelligence you’ve always wanted—delivered in a way anyone can use.
Promoting organizational adaptability and innovation
Organizational adaptability is crucial to keep pace with AI’s rapid evolution. Companies must promote a culture of innovation and continuous learning, encouraging employees to experiment with new technologies and embrace change.
For example, HR departments are increasingly leveraging AI to optimize recruitment and workforce planning, as highlighted in our Embracing the AI Revolution in HR report. By creating an environment that values adaptability, organizations can integrate AI solutions more effectively and remain competitive in a fast-changing landscape.
Cultivating AI-focused organizational structures
Organizations can better integrate AI by establishing structures that promote human-AI collaboration among AI developers, business leaders, and decision-makers. Cross-functional teams that include technical and strategic stakeholders are essential for aligning AI projects with business goals.
Appointing AI champions – leaders who advocate for AI adoption and guide its implementation – can drive innovation and bridge gaps between technical teams and decision-makers. For example, having a chief AI officer can streamline AI-related initiatives and embed them into the organization's long-term strategy.
Navigating resistance to AI-driven change
Resistance to AI adoption often stems from employee concerns about job displacement and unfamiliarity with new technologies. Leadership plays a critical role in overcoming this resistance by communicating the benefits of AI clearly and transparently.
Providing training programs in the use of AI can help alleviate fears and increase acceptance. For instance, leaders can highlight how AI tools enhance productivity for particular job positions rather than replacing them, creating a narrative that focuses on augmentation rather than a substitution by automation.
Boosting investments in AI research and development initiatives
Investment in AI research and development is essential for driving innovation and addressing societal challenges. Governments, businesses, and academic institutions must collaborate to fund AI initiatives that tackle critical societal issues such as climate change, healthcare, and education.
For example, public-private partnerships can pool resources to develop AI-powered solutions for renewable energy optimization or personalized medical treatments. Increasing funding for R&D keeps AI technologies at the forefront of innovation while addressing critical global needs.
Keep pace with AI in 2025
As AI advances in 2025, its successful adoption hinges on addressing AI challenges like the ones described in this article. With tools like Workhuman's AI-powered platform, innovation in the workplace is attainable, and it is up to us to embed ethics into its design and be transparent at all stages to all stakeholders.
It's possible to align innovation with societal trust. Proactive efforts in training, governance, and fairness through robust frameworks will shape AI into a transformative force for good.
As AI integrates deeper into our lives, will we rise to the challenge of shaping it responsibly, or will we allow its rapid growth to outpace our ability to manage its impact on humanity?